Yu Gui
Welcome to my homepage!
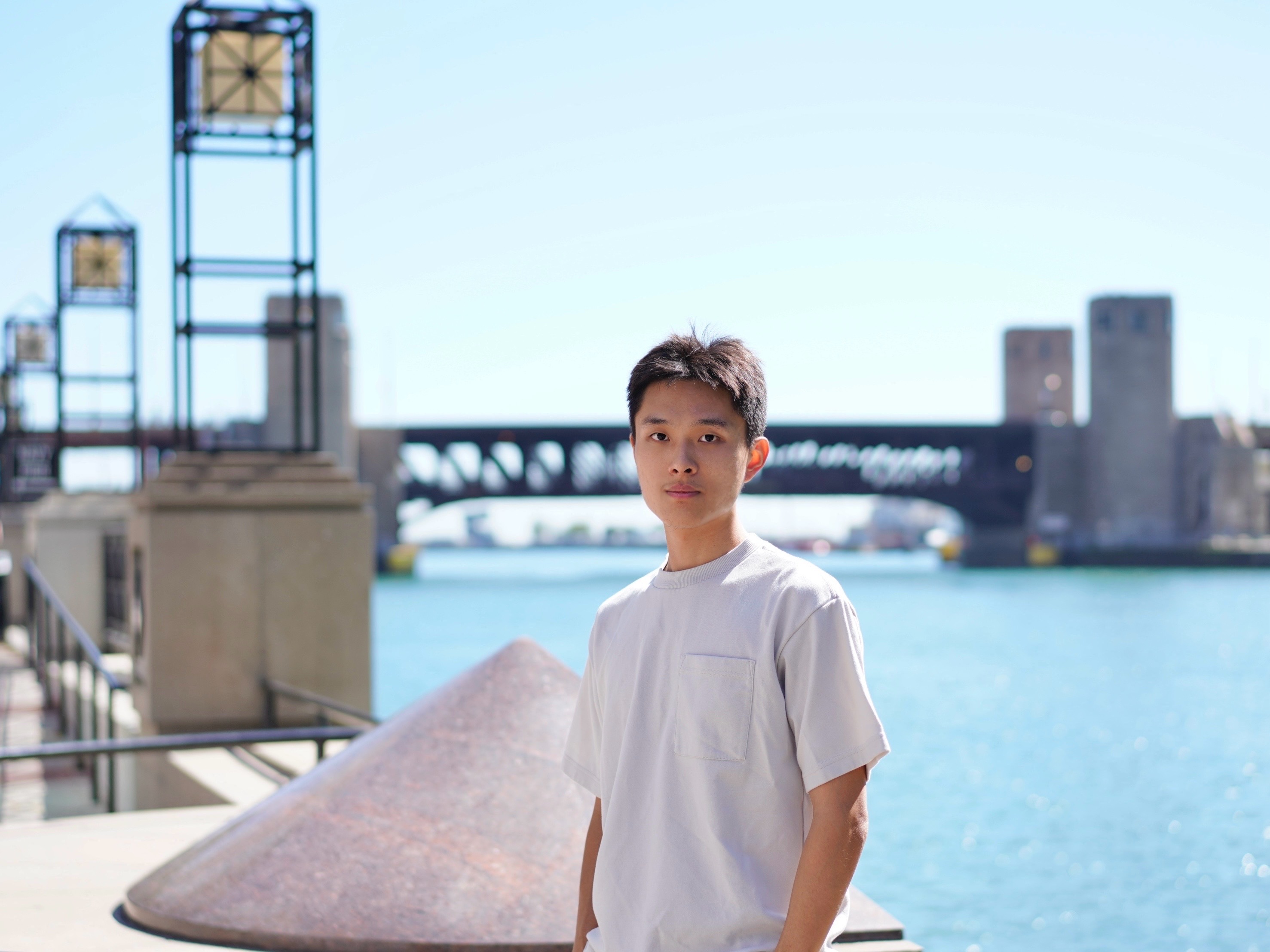
I am a PhD candidate in the Department of Statistics at the University of Chicago. I am fortunate to be advised by Professor Rina Foygel Barber and Professor Cong Ma.
My research broadly focuses on theory and methodology in scenarios where the distribution is weakly specified or fully supervised data is unavailable.
Prior to PhD, I graduated from School of the Gifted Young at University of Science and Technology of China and was a student research intern advised by Professor Jun S Liu at Harvard.
news
May 18, 2025 | New preprint: multi-modal contrastive learning adapts to intrinsic dimensions presents a theoretical analysis of CLIP and its ability to adapt to the intrinsic dimension of multimodal data enabled by temperature optimization. |
---|---|
Apr 08, 2025 | I’ve passed my PhD defense! Starting in July 2025, I’ll be working as a postdoctoral researcher in the Department of Statistics and Data Science at the Wharton School, working with Professors Dylan Small and Zhimei Ren. |
Apr 02, 2025 | Our paper Conformal Prediction: A Data Perspective has been accepted to ACM Computing Surveys. |
Sep 26, 2024 | Our paper Conformal Alignment has been accepted to NeurIPS 2024! This paper guarantees the safe and reliable deployment of foundation model outputs. |
Jul 10, 2024 | A new preprint iso-DRL on how to utilize side information (e.g. shape constraints) to balance the misspecification of sample reweighting and the over-pessimism of distributionally robust learning! As an application, iso-DRL suggests a robust approach to calibrate estimated density ratios in reweighting approaches. |
Jun 30, 2024 | I’m thrilled to be awarded the William Rainey Harper Dissertation Fellowship! |
Dec 31, 2023 | Presented conformalized matrix completion at NeurIPS 2023 and ICSDS 2023. |
Mar 30, 2023 | Received IMS Hannan graduate student award for our work on theory of contrastive learning. |